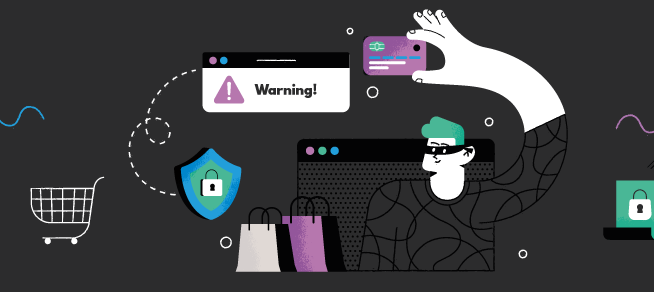
eCommerce Fraud Prevention. Detect eCommerce Fraud With Machine Learning
Inside this article:
Online shopping has lately gained even more popularity as people become more comfortable with buying items over the internet. That has led to a massive increase in eCommerce transactions, with which comes the risk of fraud. Fraudsters are always looking for new ways to exploit the system, so online merchants need to stay vigilant to protect their businesses.
In this article, we will discuss different types of eCommerce fraud and how you can use machine learning models to detect and prevent them.
What Is eCommerce Fraud, and How Does It Work
For over two decades, online shopping has been gradually gaining popularity worldwide, becoming more manageable and accessible. Its true boom, however, came with the pandemic. eCommerce has never stopped growing ever since, making retailers redefine their approach toward sales. That proves that we just needed a little push to change our habits that would likely change anyway with time.
The instant switch we experienced had both positive and negative implications. On the one hand, the retailers gained an opportunity to reduce costs related to maintaining their physical locations and reach wider target groups. On the other, with new platforms and eCommerce sales channels emerging, the fraudsters gain more opportunities to take advantage of online users.
Harness the full potential of AI for your business
Sign up for newsletterEcommerce fraud is any fraud related to online sales. Since the e-commerce industry generates numerous transactions, fraudsters have a lot of room to prove themselves. In this article, we will take a closer look at their attempts and explain how fraud detection systems can detect or prevent them.
The Different Types of eCommerce Fraud
Generally, frauds may fall into one of two categories: those which affect customers and those which affect businesses. Of course, in the case of the first category, the company will also feel the secondary effects. Some frauds, however, are specifically targeted at them. Within the scope, we can distinguish various types of e-commerce fraud with entirely different specifics. Let’s dive into the most common cases to understand the techniques fraudsters use.
Credit Card Fraud
If we had to pick one type that would deserve the infamous name of the “king of the fraud,” it would definitely be the credit card one. Even though it happens relatively rarely, considering the number of online credit card transactions carried out every day, the efficiency of the fraudsters is still worrying.
According to the Federal Trade Commission report from 2020, credit card fraud is the most common type of identity theft, making up over 20% of such crimes. Nowadays, when users regularly shop online and often have their credit card details embedded in their accounts on the shopping platforms, they’re particularly vulnerable to this type of fraud.
Credit card fraud can be understood in two ways:
- as a takeover of someone’s card details to make purchases or withdraw funds from the account
- as applying for a credit card with someone else’s details
While the second type of fraud requires a lot of “work” (the fraudster has to come into possession of various data and documents like, for instance, income records), the first one is relatively easy to carry out.
In the past, when online purchases weren’t that popular, the most common way to steal credit card info was using an ATM skimmer – a device that would gather the details after the card was inserted.
Today, it’s enough that the user makes a purchase when connected to public wi-fi. Such networks are a paradise for hackers since they make intercepting customer data extremely easy due to their architecture. The users can also fall into the trap of connecting to rogue hotspots that mimic the regular public networks but are created to steal personal data.
Fraudsters may also intercept the credit card details with phishing e-mails or pishing websites that are faithful copies of legitimate platforms that process transactions. Some also use spyware or rely on data leaks.
Friendly Fraud / Chargeback Fraud
The chargebacks have been becoming increasingly popular in recent years, and they are undoubtedly an empowering tool for customers to fight against unauthorized use of their credit cards. However, their increasing availability creates the potential for abuse.
Friendly fraud, alternatively called chargeback fraud, occurs when a customer makes an online purchase with their credit card and then demands the chargeback from its issuer. The name of this fraud is misleading, but it didn’t come from anywhere. On the surface, it may look like a legit claim, but in the end, the customer may use it to unduly withdraw from the purchase or keep the product without paying. Usually, when asking for a chargeback, the customers use one of the following justifications:
- the product didn’t arrive
- the product didn’t fulfill their expectations
- the product was purchased by an unauthorized party
- the product was purchased with a stolen credit card
- they got overcharged
The frauds of this kind can be problematic since it may be difficult for the company to prove that the claim is fraudulent. Some arguments, like the one regarding the lack of delivery, are easy to undermine, but others can cause some issues. Detecting friendly fraud is equally challenging. However, modern fraud detection systems can find patterns in customer history that would be hard to identify otherwise and link them to fraudulent activity.
Account Takeover Fraud
In the case of online e-commerce platforms, account takeovers are the kind of fraud that can cause the most severe consequences. If the user has kept automatically saved payment data, the fraudsters can use them to withdraw funds from the bank account. They also may access other vulnerable information such as personal data, addresses, company data, etc.
To take over the accounts, fraudsters tend to use similar methods as in the case of credit card details theft. They either take advantage of the insecure public networks, install spyware, or use pishing strategies, posing as customer agents of the platform or an eCommerce store. They may also create mirror-like pishing websites that retrieve account details without the user noticing.
Return Fraud
Return fraud is similar to friendly fraud - however, it doesn’t usually involve the financial institutions that have processed the transaction. Instead, the shopper claims directly to the online shop or platform that they want to return the purchased item and receive a refund. The return frauds may adopt different forms, from treating purchases as a rental (common in the clothing industry), claiming that the goods were never delivered, to returning empty boxes or removing valuable parts before returning the goods. Return fraud is often combined with credit card fraud – the fraudster uses stolen details, fresh emails, and store accounts to purchase something that they later return. Identifying some of the website behavior patterns and mixing them with external data sources may help to discover return frauds.
Gift Card Fraud
This type of fraud has gained recognition with the Google Play Gift Card scam that the scammers in the US would notoriously use for quite some time. They would call people posing as governmental agents and remind them about, for instance, fiscal obligations requiring immediate payments. The victims would be asked to transfer the money to the gift cards and share their details.
Contrary to credit card fraud, this scam is difficult to reverse once the money from the card is spent. There is no chargeback option in such a case, and the transaction will not be that easy to flag as the purchase itself is not associated with the scammed customer. However, sophisticated fraud detection models can handle that challenge, too!
Shipping and Handling Fraud
There are two ways in which shipping fraud can be understood – first, as a falsification of the shipping address, and second, as processing the purchase but never proceeding to deliver it. In the first case, different billing and shipping address is often an indicator of a scam.
The other one is a domain of the fraudulent freight forwarders. Even though it’s easy to detect, it may be hard to prove since the deliveries can be falsified in the system. Fraudsters may, for instance, attribute fake tracking numbers to the orders so that the customer cannot follow them, refuse to issue the bill of lading, or mislead clients with their vague order execution policy. The shipping fraud can harm both customers and the retailer since it affects the company’s image. Plus, the intermediary character of this fraud makes it harder to find the culprit and solve the issue. Plus, as it often takes place on international grounds, law enforcement becomes more complicated.
Reseller Fraud
This fraud has intensified with the popularization of big online marketplaces such as Amazon or Aliexpress. In a nutshell, it’s a practice of buying the product in high demand in large quantities, usually with the support of bots, and then reselling them online. The issue gained visibility during the pandemic, when the resellers would purchase bulk quantities of antibacterial gel and face masks, selling them later with an extremely high margin.
Reseller fraud is relatively easy to detect but difficult to combat. Often it’s considered a victimless crime since, in the end, the transactions themselves are legit, and lifting the margin is not against the law. However, it can affect the company’s image and its profit.
eCommerce Fraud Prevention Tools
Both e-commerce platforms and financial institutions that process the transactions support the retailers with their fraud detection systems to prevent and detect fraud. Once they identify the suspicious activity, they can either flag it and inform the retailer or take independent steps to block the fraudulent transactions from being processed.
By implementing good practices, such as strong password requirements and verifying the billing data with the AVS (address verification system), the companies can reduce the probability of account takeovers and credit card detail theft. However, it is not enough to keep their customers safe, considering the intensifying activity of fraudsters in the e-commerce field.
Some eCommerce merchants invest in the plugins or tools available in the SaaS model. These, however, may compromise safety to a certain extent since they are designed to serve any company from any field. By picking a custom fraud prevention solution, they can adjust the model to the specifics of their particular niche and its most common risks.
How Is Machine Learning Being Used for eCommerce Fraud Prevention?
Machine learning helps the fraud detection and prevention system users adjust to the dynamic nature of the fraudulent activity. The fraudsters naturally tend to change their patterns quite often, and that’s why rule-based programming keeps them one step behind. In traditional detection systems, the rules are reverse-engineered based on the occurring fraudulent incidents. That means that anytime a new type of fraud emerges, the system requires redesigning.
Machine learning systems already support retail, eCommerce, and marketing sectors with various solutions for natural language processing (NLP). Many fraud detection systems rely on analyzing documents and textual data, which are hard to process without a dedicated NLP model.
Also, the standards of the industry and every particular eCommerce website (for instance, average cart value) change, making the rules irrelevant with time. That’s not the case with machine learning, where the model establishes its own logic and identifies patterns that would often be undetectable otherwise.
Let’s give an example. We have a female American customer that travels often, and their purchase history is quite international - however, it’s always the EU country. This time, their order comes from a nearby country that is not an EU member and has a relatively strict visa policy, particularly for American citizens. That could be the hint that the transaction is fraudulent.
Aside from the location, the model analyses the user’s shopping history to check whether the purchase shows signs of unusual character. It turns out that it is a type of product the user would never buy before, and its cost is much more than the average spendings. Also, it is male-targeted. With that information, the ML model proceeds to analyze additional variables through the prism of patterns identified in the learning process until it can decide on whether to issue an alert.
Production ML fraud detection and prevention models usually analyze a wide range of data. During the initial model development process, we try to investigate all the available data. The data that is used depends on what is available and what the company wants us to focus on. In many cases, we may use data from social media lookup, attribution data, behavior analytics, logistics provider data, and customer complaints but also external data sources that will give us information on the particular customer to highlight any fraudulent behaviors and assign a preventive process whenever possible.
The Benefits of Using Machine Learning for eCommerce Fraud Detection
With machine learning, it is less likely for the retailers to miss the often subtle signs that indicate fraudulent activity. In e-commerce frauds, there is rarely any big red flag that would discredit a suspicious customer. It’s rather a combination of variables. The machine learning model can use what it has learned about the particular customer and the purchase patterns in the online shop to find that little deviation from the norm.
Machine learning models for e-commerce fraud detection help retailers reduce the number of false alerts since their accuracy is much higher. Traditional systems often make the legitimate customers migrate to other online store as it affects their user journey negatively. Also, by engaging artificial intelligence-based systems, the retailers can reduce the size of the fraud analyst team, cutting costs significantly. Of course – machine learning models still may need some verification mechanisms, but they reduce the amount of engagement required, unlocking funds for investments in the other fields.
The Future of eCommerce Fraud Prevention
Fraud prevention will likely become even more machine learning-dependent in the future. Lately, it has become increasingly common to apply deep learning techniques to detect fraudulent activity. When it comes to fraud protection, the more data, the better – and neural networks are the best at processing them effectively. Combined, supervised, and unsupervised deep learning methods enable companies to detect fraud in real-time, significantly reducing fraud-related harm. We also notice a strong migration towards custom detection systems that make accurate feature extraction and pattern identification easier.
Contact us today and learn how you can prevent fraud with a custom machine learning system for eCommerce fraud detection.