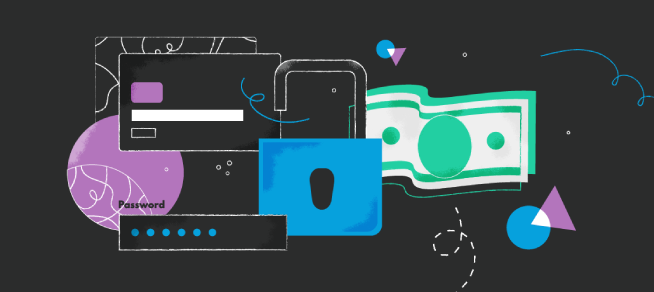
AI-Based Fraud Detection in Banking and Fintech: Use Cases and Benefits
Inside this article:
The fraud detection process in the banking and fintech sectors has always been a challenging task for analysts. Fraudsters are becoming more sophisticated day by day, and it is becoming increasingly difficult to identify fraudulent activities without using artificial intelligence (AI) techniques.
In this article, we will discuss different use cases of fraud in the banking and fintech sectors and explore the benefits of using machine learning solutions for fraud detection. Stay tuned!
What Is Fraud Detection, and Why Is It Important?
Fraud detection is a process of identifying fraudulent actions and scams. Most of the time, they involve attempts to obtain financial resources under false pretenses. However, some frauds do not fall under this category – like hacker attacks that aim to strain the company’s image rather than obtain financial benefits. The frauds can undermine the entire foundation of the business, causing both financial and image losses. Thus, their prevention is essential for companies across different sectors.
The techniques that enable detecting frauds can slightly differ depending on the case. Not that long ago, the whole responsibility would lie on the analysts and specialists with a background in risk assessments. Manual verification of the operations is labor-intensive and time-consuming, though. With the digitalization of various processes, the effectiveness of the manual approach has dropped, particularly in operation-intensive industries such as banking.
The technological advance, fortunately, provided the business owners with a remedy – automation. AI-driven tools today constitute a crucial part of fraud detection procedures, supporting manual verification or even replacing it entirely. The fraud schemes are becoming increasingly complex and sophisticated, but the development of machine learning techniques goes hand in hand, providing the companies with the ability to defend themselves. In this article, we’ll look deeper into these empowering methods, analyzing their requirements and effectiveness in particular industries. You can read about our case studies about auto insurance fraud claim detection here.
How Do Fraudsters Operate, and What Are the Different Types of Fraud?
The frauds themselves may be of different nature. Some involve identity theft or illegal takeovers, which usually result from organized criminal activity and require some tools and industry insights. Other, less severe ones are more of stretching the truth, for instance, when faking data in a loan application. Even if they do not have the power to affect the company’s image, they may directly impact its financial safety.
Fraudsters operate either in organized groups or on their own. The groups are the hardest to fight with since the accumulated insights and dynamically changing strategies make their actions very effective. There’s also a distinct category of fraudsters acting both alone and in groups – the hackers. In their case, the purpose is often different from personal benefit. Regardless of the reason, hackers’ attempts require a different approach than the typical one-person frauds since they can compromise its market position within a few minutes.
One of the most common kinds of fraud today is phishing – a method of obtaining sensitive data like login information, passwords, or identification details via mail or SMS. Phishing fraudsters usually pretend to send these messages in the name of well-known, respected companies. They often use almost identical domains, which makes it extremely easy to fall into such a trap. In some cases, the fraudsters operate on fake websites that look exactly like the original one, pishing for the credit card details. The already well-known fake Airbnb site scam is a good example.
Fraudsters also steal identities in order to access financial benefits – for example, get a loan regardless of their insolvency. In other cases, they may use their real identity but conceal their bad credit history or deliver fake documents to obtain a better credit score.
The Benefits of Using AI for Fraud Detection
With advanced analytics, financial institutions and fintech companies predict the possibility of frauds in order to avoid them before they even occur. Simultaneously, they can scan the operations for the traces of fraudulent activities. If they detect fraud, they are able to immediately flag suspicious activity and take further steps, either manually or with the help of automation tools. By engaging the machine learning techniques instead of relying on a rule-based approach, they gain the capability of detecting the often subtle correlations between customer behaviors and fraudulent potential.
Artificial intelligence provides them with incomparable security, too. Using fraud detection systems, they can minimize the risk of financial loss to which the frauds expose them. Besides preventing the actual frauds, they’re able to reduce the probability of tying the business knot with the risky partners and identify the illegitimate customers. AI helps them see the big picture when evaluating applications, documents, and inquiries.
It’s not only about the increased processing capacity. Taught with extensive datasets, the machine learning algorithms are able to flag a risk-implying combination of variables that would not be alarming to the human eye. There’s no necessity to reverse-engineer the rules based on fraudster’s behaviors, since neural networks can foresee them.
Another great benefit is the efficiency increase. The faster you can act, the more chances are of minimizing the severity of damage the fraud will cause. Artificial intelligence automates data processing, taking away the pressure of manual evaluation which is less likely to be efficient with the information in large quantities. The specialists can meanwhile focus on solving more complex cases and diving deeper into each instead of trying to deal with tons of data.
Efficient AI-based fraud prevention systems also uphold the financial firm’s image. Many kinds of frauds affect directly not only the businesses but also their customers. The clients who have suffered from the repercussions of the fraudulent operations may migrate to other companies. The reputation of a “fraud-prone” may also keep the new customers from committing. With fraud detection, businesses can maintain customer trust, protecting themselves from image losses
Case Studies of How AI-Based Fraud Detection Has Helped Banks and Other Organizations
Fraud detection is widely applied in banking and fintech, but the demand for it is also increasing in other industries, such as insurance or healthcare. Governmental structures such as tax offices also use it to streamline their operations and take the time pressure off the employees dealing with data-intensive tasks on a daily basis.
How does that work in practice? What are the specific uses of this artificial intelligence-based technology, and which mechanisms is it supported by? In order to illustrate it, we’ve gathered some use cases with real-life examples - some based on our own experience.
Credit Card Fraud Detection and Identifying Fraudulent Transactions
There are many ways for the customers to protect their credit card details or account data – for instance, using virtual private networks and virtual cards or verifying the website certificates. However, with the fraud techniques becoming increasingly elaborate, it is essential for the entities processing the credit card transactions and transfers to scan them in order to prevent the danger from occurring.
In order to teach the model to recognize the fraudulent activity, it needs to be fed with an extensive dataset containing both types of transactions. Analyzing it, the model learns to recognize the indicators of fraud. What can make it flag the transaction? For instance, a sudden increase in weekly or monthly transaction values on the customer account, a purchase in a shop that does not deliver to the country of account holder residency, or an atypical expense. These anomalies divide into point, collective, and conditional.
Discovering Identity Theft
Credit card fraud can be one of the subcategories of identity theft, but there are others, too. With an increasing digitalization of processes – such as opening accounts, submitting applications, or tax declarations – we are becoming increasingly vulnerable to scams in this area. Digitalized data is easier to reach out for, creating identity theft opportunities for fraudsters. They can use the stolen identity details to open accounts in someone’s name, access another person’s insurance or other benefits, or even steal their tax return.
Fortunately, the AI-driven detection systems for identity theft are very effective at minimizing the risk of such frauds and detecting them at an early stage. Depending on the case, the models may detect the suspicious transactions or behaviors that do not match the customer’s behavioral patterns or doubtful information in the provided documents, preventing the potential threat.
Loan and Mortgage Application Fraud Detection
Banks use fraud detection systems extensively in order to evaluate the loan and mortgage applications with regard to the potential fraud. It’s an essential element of their risk assessment that supports the analysts in their daily work. They can extract relevant information from the applications with the help of the NLP and then evaluate them with a model trained with the dataset containing both correct applications and these recognized as fraudulent.
The model identifies patterns that may indicate potential fraud – for instance, a big gap between annual gross income in two subsequent years paired with specific demographic data. Of course, it may cause false alarms – it’s the analyst’s job to evaluate that further.
You can read more about the main challenges of building such a loan application fraud detection system in our case study
Related case study: Loan Application Fraud DetectionA company from the finance sector approached us with a significant problem - they wanted to develop an AI model that could help them make smarter underwriting decisions and faster approval process by automatical identification of fraudulent loan applications.Our challenge? Identifying fraudulent loan applications manually is challenging because of the substantial volume of cases to be reviewed each day. Fraudulent applications take a long time to detect and even longer to deny, resulting in more significant revenue loss. The challenge was to create a predictive analytics model that could sift through all loan applications and sort out the probable fraudulent ones from the genuine ones within minutes. Read more about this case study.
At the same time, advanced AI allows the banks to estimate the likelihood of committing fraud by a particular customer. By analyzing the customer behavior data (financial transactions, account history, loan history, etc.), the deep learning model is able to predict the probability of such tendencies.
Uncovering Money Laundering Activities
Deep learning models help the banks find hidden correlations between account activity and criminal activity. Money laundering is exceptionally hard to detect with traditional methods, ass the factors pointing to it tend to be really subtle. Since such a practice is usually a domain of organized criminal groups or seemingly legitimate companies and involves big money, every move is carefully thought-out.
But slip-ups happen to everyone – it’s just hard to detect them between the cover-up transactions that do not raise any suspicion. It could be one transfer that exposes the whole scam. Sometimes, however, it’s a combination of movements on the account that look legit separately, but not when put together. The AI can immediately flag these patterns, which could otherwise remain noticeable for the expert’s eye.
What to Consider When Developing an AI-Driven Strategy for Financial and Banking Fraud Analytics?
For the AI-driven strategy to be efficient, the developers and data scientists developing the fraud detection model should pay attention to a few essential factors.
The Dataset
The main problem that developers and data scientists face, regardless of the nature of the fraud the model is trained to detect, is the imbalance of the dataset. In order to learn to distinguish the fraudulent and genuine operations, the model needs to have enough examples to draw the patterns from. That’s challenging considering the ratio of the positive and negative classes. The frauds are relatively rare in the sea of genuine transactions – it’s obviously something good, but not in terms of machine learning model training.
That’s why, in order to learn effectively, the model requires a large-sized and good-quality dataset. The complexity of the model itself has to increase as well, as a simple one will not be able to process all this information with satisfactory results.
Another dataset-related issue to face is confidentiality. The financial institutions are not eager to share their datasets, even if it’s for training purposes, since it may lead to confidential data leaks. Even if using its database, the entity may deal with confidentiality issues as the data concerns its customers directly.
The Dynamically Changing Environment
Fraudsters are not easy to deal with as they adapt very fast and change their strategies at a fast pace to maximize the chances of success. That forces the companies to retrain their fraud detection algorithms every once in a while so that they can easily identify emerging patterns that wouldn’t be detectable in previously provided datasets. The good thing is that it’s hard to invent something new in this area, so a well-trained machine learning model can use its previously gained knowledge to detect suspicious activity even if the fraudsters modify their “style” over time.
The Future of Fraud Detection and How AI Will Help to Prevent a More Sophisticated Future of Banking and Fintech
So far, fraud detection is not yet dominated by the machine learning approach, since the banks and fintech sometimes consider rule-based systems safer and easier to implement.
At this point, the two approaches tend to complement each other. However, it is likely to change in the nearest future. That’s because rule-based systems’ complexity has its limits, and also because the fraud attempts are becoming increasingly sophisticated and change more dynamically than in the past. Considering that the rule-based approach requires creating new rules every time new patterns emerge, it’s a losing battle for the banks and other financial institutions or companies. With the machine learning approach, they can actively predict the fraud instead of always staying one step back.
The advance of deep learning techniques helps financial institutions create a safer environment for their customers while protecting their own financial integrity. We’re happy we can be a part of that process!
If you would like to use AI for the purposes of a fraud detection system or consult your specific case, feel free to drop us a line.