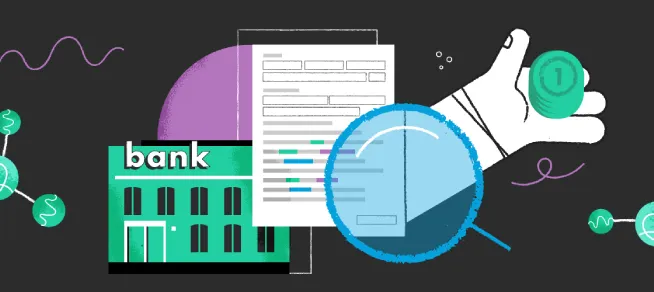
NLP in Banking, Fintech, and Insurance. How Insurance and Financial Institutions Can Implement Natural Language Processing?
Inside this article:
The insurance and financial services industries are prime candidates for natural language processing (NLP) technology. NLP can help banks, insurers, and other financial institutions automate processes, improve customer service, and make better decisions.
In this article, we will explore how NLP can be used in finance and insurance and discuss some of the challenges that need to be overcome when implementing NLP in business.
NLP Technology
Natural Language Processing is a branch of computer science that, in a nutshell, aims at teaching computers to comprehend human language. It’s not a new concept - its roots date back to the WWII times. However, only after the popularization of artificial intelligence in the first decade of the XXI century, it has started playing a significant role in our everyday life.
Today, NLP finds various applications across different channels. Anytime you translate text in your browser, you take advantage of this technology. It’s the same about asking Alexa about the weather forecast or discussing the details of your canceled flight with the chatbot.
Although NLP is often called a branch of machine learning, in fact, these are two subbranches of AI that complement each other. Without machine learning algorithms, computers wouldn’t have the ability to get better at understanding language with time and practice, as happens with humans. That’s why these two make an inseparable duo.
Harness the full potential of AI for your business
Sign up for newsletterBut how does the machine actually make sense of what we say or write? The whole process starts by breaking down the elements of the text or recording them into tokens during the phase called tokenization. Once they are identified, the NLP tries to find relationships between them on different levels: syntactic, semantic, morphological, discourse, and pragmatic.
What’s worth mentioning is that Natural Language Processing may involve both understanding (NLU) and generation (NLG). The second category brings great benefits to the customer service sector due to its interaction-enabling qualities. Natural language generation also serves for such features as spelling autocorrection and automatic e-mail response.
Today, we will focus on other sectors that have been discovering NLP for themselves in recent years - banking, finance, and insurance. If would like to delve deep into the concept of NLP first, check out our definitive guide to this technology that explains its intricacies to the smallest detail.
Unstructured Data in the Insurance and Financial Industry
Just as the enterprises from other sectors, insurance companies, and the financial sector operate both on structured and unstructured data. In their case, the percentage of structured data may be actually higher than in other industries since a big part of their processes (like customer acquisition, applications, and detailed analytics) are standardized and formalized.
However, let’s not forget that these sectors are also known for their affection for paperwork - and that means a lot of documents to process. These, as well as e-mails, legal reports, contracts, videos, recordings, and so on, fall under the category of unstructured data. Such data is more difficult to process since it hasn’t been put through any standardized process of capturing (like online forms or surveys).
Whereas the databases or spreadsheets contain only relevant and categorized information, in their case, the insight is hidden between words. While it may be easy to find it for a human, the computer needs some support to understand what these resources contain. On the other hand, the insurance and finance companies need their computational power since dealing with a ton of documents is counter-effective without automation.
If processed thoroughly, such unstructured resources can bring powerful insights that protect their financial security, boost profit and improve customer satisfaction. However, due to their unstructured nature, they are incomprehensible to the computer. Fortunately, there’s a solution to that issue - and it’s the subject of our today’s article.
Natural Language Processing Applications
Natural language processing helps companies from the insurance and financial services industry find relevant information across unstructured resources. That can be particularly helpful in situations requiring rapid knowledge transfer, like company fusions. It’s also a blessing for the companies that sign long-term contracts with many annexes throughout the years. With NLP, they can keep track of changes and updates and follow the settlements made via such channels as e-mails or calls, which are also legally binding.
NLP in Banking and Fintech
Let’s first take a closer look at each of the most popular applications of NLP in the banking industry to understand why these sectors have embraced it so tightly in recent years.
Sentiment Analysis
We will start with one of the most universal NLP-based tools across industries, particularly now, when the pressure on customer satisfaction has increased dramatically. Sentiment analysis is a field of Natural Language Processing that enables computer software to understand human emotion based on provided content. After breaking down the unstructured content - in this case, a written review - into tokens, the trained algorithm links them and their combinations with a particular emotion.
With this method, the companies can gather insights from the reviews of the apps and services left on the company’s website, e-mails, and testimonials across different channels. The numeric grade system or other scales tend to be quite limiting, not providing the company with much useful information. Using NLP, they can make the most out of the available customer data. Processed this way, the user content can serve for advanced analytics in the field of customer satisfaction.
Risk Assessment
Banking is a risky business. No one likes being a subject of the time-consuming underwriting processes, but the truth is, every accepted loan application is a risk for the financial firms. However, it can be reduced to the minimum with NLP techniques. The banks can extract the relevant data from the documents provided by the candidate via named entity recognition and assess their profile in terms of loan risk based on the established criteria and the insights gained via machine learning. Aside from the data from the application documents, the model can include the account history and credit history, as well as other historical data. This way, the analysts get an even bigger picture.
Loan Application Fraud Detection
As a sector that bears big responsibility and risk, banking requires constant improvement of the fraud detection techniques. These are becoming increasingly sophisticated and difficult to pick up as a result, particularly with the substantial volume of applications waiting to be reviewed.
NLP algorithms can take the pressure on the fraud detection department, partially automating the process of reviewing loan applications. With its help, the banks can identify the relevant information in the provided documents. Depending on the case, it may be account activity history, credit history, loan transaction details, income, etc. This data is later evaluated by the machine learning model (most likely logistic regression) trained with historical records of genuine and fraudulent applications.
We have created such a model as a part of one of our projects. If you would like to read more about this particular use case of NLP-powered software, here’s the case study.
Virtual and Voice Assistants
The demand for customer services in the banking and fintech sector is high. However, the range of problems in the inquiries is actually quite narrow. Since they are so repetitive, virtual assistants are a perfect solution to streamline problem-solving and reduce customer service costs.
With NLP, machines extract the information from the user’s writing or speech in real-time and generate the relevant answers. Chatbots or voice assistants based on deep neural networks can engage in quite natural interaction with the client and learn with each such exchange to improve the accuracy of the answers. Such models may include sentiment analysis to improve the quality of the “conversation”.
NLP in Finance Sector
Document Classification
When carrying out a loan audit, the financial institutions need to go through an extensive set of documents (usually scanned), among which there are forms, surveys, and so on. Each type of document has a different structure and specifics. In order to facilitate successful data extraction and improve its accuracy, it’s worth first carrying out document classification with NLP. The algorithm can identify the elements of the content associated with a particular type of document and classify it to save the audit specialists and data scientists’ manual work.
Financial Sentiment
The financial landscape is driven by sentiment - a drop in trust can cause a chain reaction that will shake the whole economy. That’s what happened recently to Terra (LUNA), the fall of which has questioned the future of the crypto market. No wonder financial institutions want to have those sentiments under control.
In order to do so, they employ the NLP for the purpose of extracting the relevant information from financial articles as well as tweets, social media posts, and stock market opinions on StockTwits. That provides their financial analysts with meaningful insights on the market moods as well as trusted and questioned investments or authorities.
Document Summarization
Without the automation tools, we have to read through the whole document to find one single piece of information we’re looking for. Same when trying to understand its core concepts.
In finance, the volume of documents makes manual processing insupportable. Fortunately, companies can use NLP models for document summarization. It enables them to condense the initial text while maintaining essential information. They can do it via two approaches - extractive or abstractive. The first one depends entirely on the original content, while the other is more complex, generating a brand-new summary after interpreting the initial text. In the case of finance applications, the first approach usually works just fine, as the summary doesn’t need to be fluent and cohesive.
Semantic Search
NLP techniques can make the financial enterprise or institution customers solve their issues faster and easier. An NLP technique called semantic search brings the traditional search to the next level by determining its intent and context instead of just relying on the keywords.
In finance, that technique can be used for supporting recommendations within the application or the website. The portfolio of the banks can be impressive, and the users will surely appreciate a little help with finding what they’re looking for. Once they get a perfectly matching recommendation, they are more likely to finalize their order. Semantic search can also improve the customer experience with chatbots and FAQs.
NLP in Insurance
Claims Processing
Effective claims processing system is the backbone of every successful insurance company. Manual workflows in this field are slowly being replaced with partial automation since it enhances productivity, but also reduces the probability of human error. In order to process a claim, the insurance agent has to look through different types of resources, all containing unstructured data.
Here’s where NLP comes to the scene. It streamlines that process, extracting relevant entities and interpreting them within the context of the document. The machine learning model trained with historical underwriting data then evaluates the extracted information, detecting potential red flags and helping the agents assess the risks related to the particular case.
Detecting Fraudulent Claims
Just as it was for loans, NLP also streamlines detecting fraudulent insurance claims. The spectrum of potential frauds is broad, from reporting nonexistent injuries, submitting claim forms for surgeries or accidents that never occurred, and falsifying healthcare reports, among others. Identifying these frauds manually is difficult, as often the devil’s in the details. Especially when the volume of the applications is high, the insurance agents responsible for verifying claims may struggle to detect red flags.
With NLP, insurance companies can easily extract information from the delivered applications and then feed it to the AI model trained with genuine and fraudulent application data. When developing our car insurance claims fraud detection system, we have picked the logistic regression model as it provided high accuracy with a relatively limited dataset (considering that fraudulent cases are relatively rare in the car insurance sector).
You can read more about the details of this project h ere.
Benefits of Applying NLP
NLP saves time and effort, enabling automation in various fields. The finance and insurance companies, for which processing tons of documents every day is daily bread, use it to reduce the amount of mundane work prone to human error. It streamlines processing applications, but also has a great impact on the quality of customer service.
By applying natural language processing in their workflows, the companies can:
- prevent financial loss - NLP helps them detect anomalies or potential fraud across applications and keep track of the market sentiment, supporting the risk management
- keep better track of their data - NLP gathers unstructured data across such channels as e-mails, contacts, applications, documents, etc.
- reduce the business risk - NLP allows them to scan through the account history, the previous applications, and other data to provide the client with the score or support the underwriting process
- improve the productivity - NLP extracts relevant information and provides summaries so that employees get better insight and focus on less repetitive tasks
- get better feedback - NLP analyses sentiment in provided reviews, e-mail, and other content
- increase customer satisfaction - NLP takes over a part of customer agent duties, solves the most common issues supporting chatbots and virtual assistants, and streamlines the ticketing system.
Recent Advancements in NLP Technology - Why It Is the Best Time to Invest in NLP Solutions?
Since it’s proven to provide multiple benefits across industries, NLP technology has been gaining momentum in recent years, and many companies consider its implementation or development a priority. Strong emphasis is being put on developing the accuracy of the NLP-based solutions in languages other than English. Some, like Polish, require much more elaborate algorithms to come up with satisfactory results due to the complexity of their inflection.
Content is still king, and the development of advanced generative techniques is a spark of hope for the enterprises that spend a big part of their budget on content creation and management. GPT-3 (3rd generation Generative Pre-trained Transformer) is a deep learning model created specifically for the purpose of text generation and a new frontier of the NLP revolution. As the largest neural network ever created, GPT-3 stands out with its capabilities to create realistic human text and deal with all the nuances of human language.
GPT-3 opens new possibilities in front of companies across different sectors, particularly in the field of content management and customer service. Companies can use it to generate articles, e-mails, notifications, and any other type of content that requires realistic human text.
If you think an NLP application could help you reach your business goals or improve the results in a particular field, let’s talk! Nexocode is a leading natural language processing development company. We have a bunch of NLP-based projects in our portfolio and would love to launch another one.
And if you’re wondering where to start - join our AI Design Sprint workshops focused on NLP to design your next AI-based solution.